Digital twins in personalized healthcare create virtual models of your body, combining genetic, clinical, and environmental data for real-time insights. They help predict disease progression, optimize treatments, and enable early interventions tailored specifically for you. Using advanced sensors, AI, and multi-scale data, these digital replicas support more precise and proactive care. If you want to understand how this technology is transforming medicine and what’s ahead, keep exploring further.
Key Takeaways
- Digital twins create virtual, personalized models of patients by integrating genetic, molecular, clinical, and environmental data for tailored care.
- They enable real-time simulation of disease progression and treatment responses to improve diagnosis and intervention strategies.
- Advanced AI and data fusion techniques enhance the accuracy and predictive capabilities of personalized healthcare digital twins.
- Implementation challenges include data interoperability, privacy concerns, high costs, and the need for robust validation and regulatory frameworks.
- Multidisciplinary collaboration and infrastructure development are essential for deploying digital twins effectively in clinical practice.
Understanding Digital Twins in Healthcare
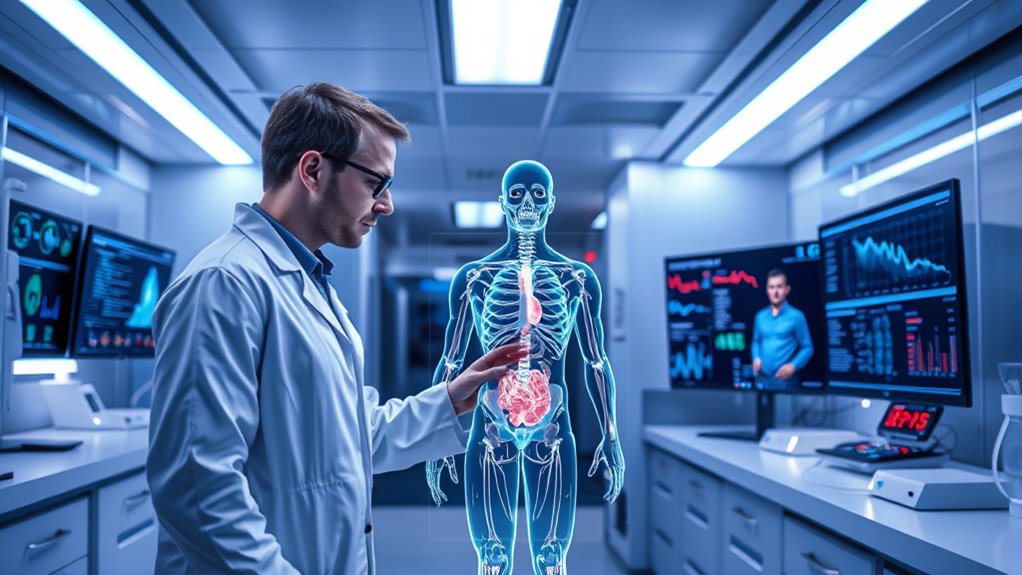
Digital twins in healthcare are virtual models that accurately replicate an individual’s biological and physiological systems. These digital twins integrate diverse health data—genetic, molecular, clinical, and environmental—to create patient-specific, dynamic representations. High fidelity is essential to ensure these models provide precise and reliable simulations. Using advanced healthcare technology, these virtual models enable real-time simulation of disease progression, treatment responses, and health trajectories. Machine learning enhances their accuracy and adaptability, allowing for personalized medicine approaches. With disease modeling and continuous data exchange, digital twins provide high-resolution insights into complex biological interactions. They support early detection, intervention planning, and drug development by offering detailed, patient-specific information. This innovative technology relies on multidisciplinary collaboration and sophisticated network modeling to produce predictive virtual counterparts that can transform healthcare outcomes. Additionally, incorporating protective styling benefits from textile applications can inspire novel approaches to patient comfort and interface designs in wearable health devices. Moreover, the integration of real-time data improves the responsiveness and relevance of these models, ensuring they stay aligned with the patient’s current health status. Furthermore, establishing robust data security measures is critical to protect sensitive health information and maintain patient trust as these technologies become more widespread. As the technology advances, the importance of data privacy and secure data management becomes increasingly critical to maintain patient trust and comply with regulations.
The Evolution of Digital Twin Technology
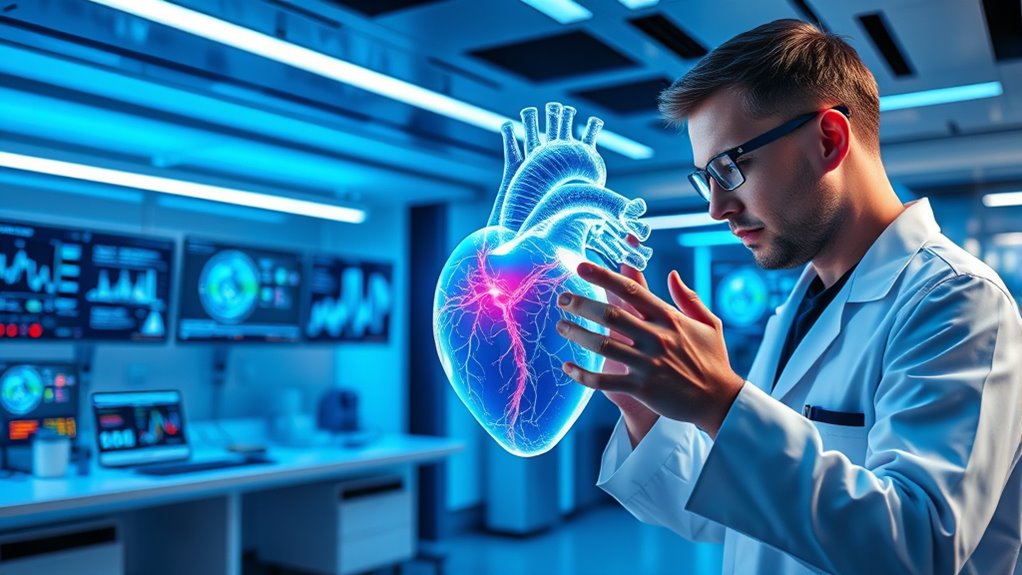
Digital twin technology started in manufacturing and aerospace, with NASA using it in the 1960s to simulate spacecraft. Over time, it has evolved into dynamic, real-time models in healthcare, integrating diverse data for personalized insights. The integration of Essential Oils and other holistic approaches is increasingly being explored within digital twin applications to enhance personalized healthcare solutions. Additionally, advancements in juice cleansing and detox strategies are inspiring more holistic approaches to health management, which could be integrated into digital health models. Incorporating home decor concepts such as creating a calming environment might also support patient well-being within digital health frameworks. Recognizing the importance of fatherhood and family bonds can also inform more compassionate and family-centered healthcare models. As the field expands, the role of grocery savings strategies in supporting patient nutrition and wellness becomes increasingly relevant.
Origins in Manufacturing
The roots of digital twin technology trace back to the 1960s when industrial manufacturers began creating virtual models to simulate and optimize products before building physical prototypes. Imagine:
- Using simulation to test product designs, reducing costly errors.
- Applying sensor technology and IoT to gather real-time data on machinery.
- Employing modeling and automation to enhance the entire product lifecycle.
- The integration of existential themes in technological development encourages innovation and a deeper understanding of human-technology interactions.
- The development of digital models that could adapt and learn over time laid the groundwork for today’s intelligent digital twins.
- Early implementations of these virtual models demonstrated the potential for real-time data integration, which is now fundamental to modern digital twin systems.
- As technology advanced, the ability to synchronize physical and virtual environments became a central feature, enabling more accurate and dynamic simulations.
These early digital twins helped streamline manufacturing, allowing engineers to predict failures and improve performance.
NASA adopted similar concepts in the 1960s to troubleshoot spacecraft systems during missions like Apollo 13.
Over time, the term “digital twin” was coined in 2005 by Michael Grieves, formalizing its role in manufacturing, where it evolved into various forms—static, mirror, shadow, and intelligent twins—powered now by advanced sensor tech and real-time data.
Advancements in Healthcare
How have healthcare providers transformed patient care through technological innovation? Digital Twin Technology now enables highly personalized healthcare by creating detailed virtual models of individual patients. These models integrate medical data from various sources, including clinical, molecular, environmental, and social factors, allowing for exhaustive disease modeling. Additionally, applying the Law of Attraction principles can help optimize patient engagement and positive health outcomes by aligning mental and emotional states with healing goals. Advances in machine learning, IoT, and high-performance computing facilitate real-time data collection and dynamic health monitoring. Early clinical applications include FDA-approved digital twins in cardiology and artificial organs like the pancreas. Incorporating biodiversity concepts, such as diverse data sources, enhances the robustness and accuracy of these models. Large-scale projects, such as Johns Hopkins’ rapid digital twin creation and Singapore’s city-scale models, demonstrate progress toward scalable, interconnected healthcare systems. These innovations support treatment optimization and preventive care, marking a significant evolution in how healthcare providers deliver personalized diagnosis and effective interventions. Furthermore, the integration of patient-specific data helps tailor interventions that improve overall health outcomes and patient satisfaction. The development of ethical frameworks ensures that these technologies are implemented responsibly, respecting patient privacy and autonomy. Moreover, fostering interdisciplinary collaboration accelerates innovation and the development of comprehensive healthcare solutions.
Components and Types of Healthcare Digital Twins
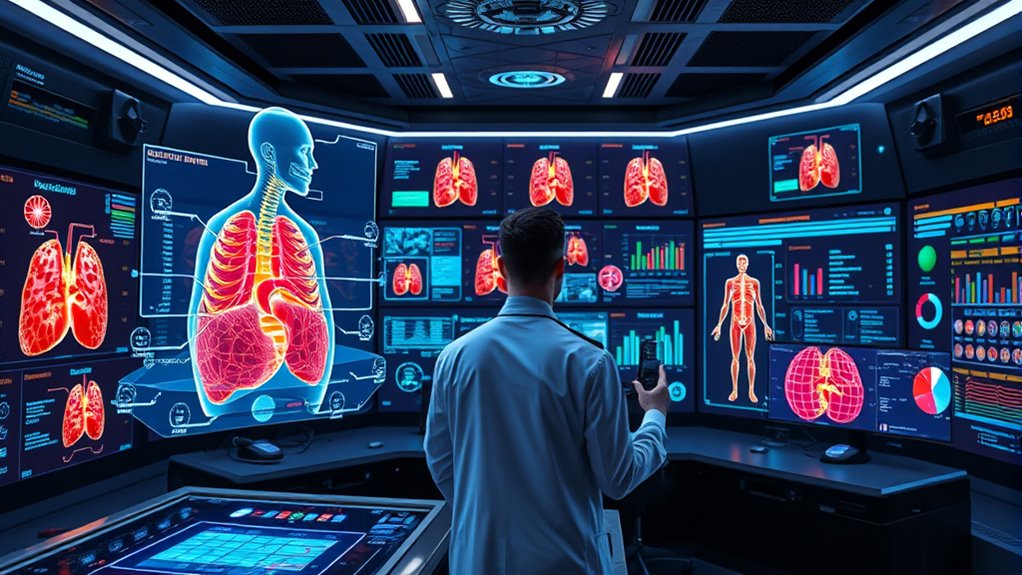
Understanding the components and types of healthcare digital twins helps you grasp how they mimic real patients or devices. These twins consist of physical entities, virtual models, and real-time data links, each serving a specific purpose. Incorporating advanced imaging techniques and real-time monitoring data enhances the accuracy and effectiveness of these digital representations.
Twin Components Overview
Healthcare digital twins are composed of three essential components that work together to create a dynamic and personalized simulation. First, the physical patient or device provides real-world data. Second, the virtual model integrates multi-scale data—clinical images, molecular profiles, environmental factors, and history—to enhance simulation accuracy. Third, a bi-directional data connection enables real-time updates, ensuring continuous interaction between the physical and virtual entities. Mastering Time Zones With Our Ultimate World Clock Tool Envision this as: 1. A virtual model mirroring a physical patient, constantly updated with bi-directional data. 2. Multi-scale data fueling dynamic prediction for personalized treatment. 3. Real-time updates refining healthcare delivery and enabling early interventions. Additionally, understanding data integration is key to creating effective digital twins that adapt to individual patient needs. Incorporating emotional support strategies can further improve patient engagement and outcomes by addressing psychological well-being. These components allow for precise, adaptive healthcare, supporting treatment optimization, early intervention, and improved outcomes through more accurate, real-time insights. Furthermore, advances in patient-specific modeling enhance the ability of digital twins to simulate complex biological processes accurately.
Types and Capabilities
The landscape of healthcare digital twins includes several distinct types, each designed to serve specific functions and levels of complexity. These digital twins create a virtual replica of a patient’s health, enabling bi-directional data flow for real-time monitoring and interaction. Types of digital twins vary from static twins, with fixed properties, to mirror twins that simulate dynamic functions, shadow twins that adapt through continuous data updates, and intelligent twins integrated with AI for autonomous reasoning. Advances like IoT sensors, machine learning, and cloud computing have expanded their capabilities. Sound healing science has also contributed insights into how physiological responses can be influenced through specific frequencies, potentially enhancing the accuracy of health simulations. Multi-layer models combine molecular, phenotypic, and environmental data, creating detailed representations for personalized healthcare. These diverse healthcare applications leverage predictive analytics and real-time insights to improve diagnosis, treatment, and patient outcomes.
Building Personalized Models Using Network Tools
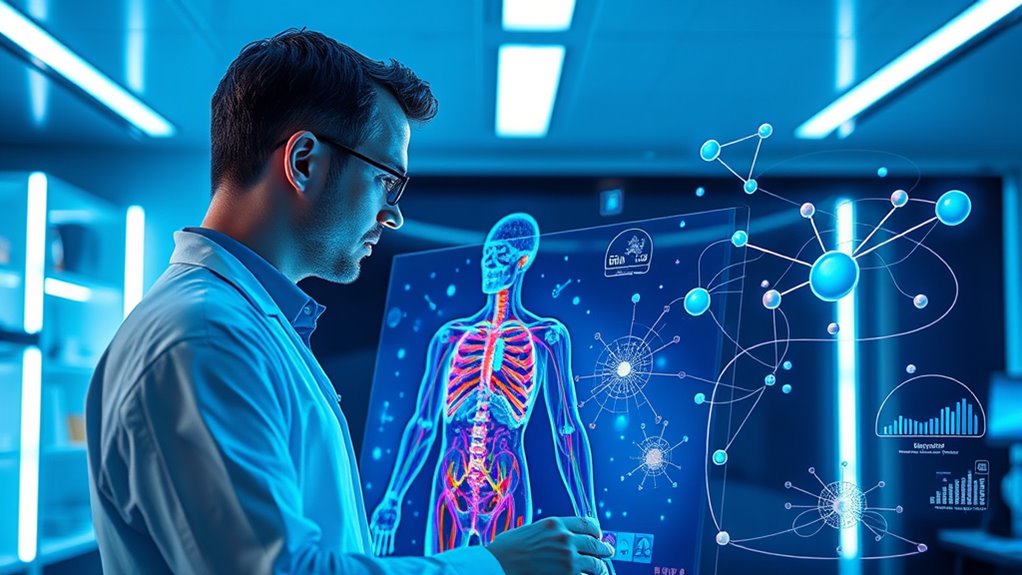
Building personalized models using network tools involves mapping disease-associated genes onto interconnected molecular networks to identify key disease modules. You can visualize this as pinpointing critical hubs in a web of molecular data, revealing how different components interact. A comprehensive understanding of biological interactions enhances the accuracy of these models by incorporating various data types and methodologies.
Mapping disease genes onto molecular networks reveals key disease modules and interactions.
Think of it like:
- Connecting genes, proteins, and pathways to form a holistic network.
- Integrating multi-omics analyses—like mRNAs and proteins—for detailed insights.
- Linking molecular, phenotypic, and environmental data to craft patient-specific models.
These network modules help prioritize biomarkers and therapeutic targets tailored to individual disease mechanisms.
Integrating Multimodal Data for Enhanced Accuracy
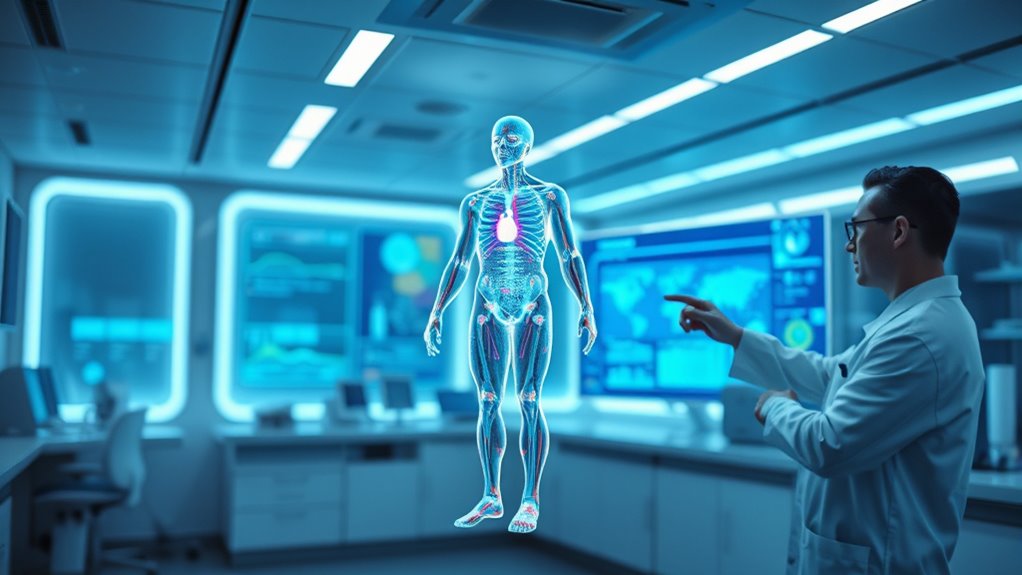
Integrating multimodal data into digital twin models markedly boosts their accuracy and ability to reflect complex biological realities. By combining clinical images, genomic profiles, molecular biomarkers, environmental factors, and patient history, you enhance the model’s depth. Multi-omics datasets—genomics, transcriptomics, proteomics, and metabolomics—capture the intricate mechanisms behind health states. Incorporating real-time monitoring from wearable sensors and electronic health records enables dynamic, adaptive health insights. Advanced data fusion techniques and machine learning harmonize these heterogeneous data sources, elevating digital twin accuracy and predictive power.
Data Type | Source | Application |
---|---|---|
Genomic profiles | Multi-omics datasets | Disease mechanisms |
Clinical images | Imaging devices | Structural insights |
Electronic health records | Healthcare providers | Real-time health updates |
Molecular biomarkers | Lab tests | Biomarker tracking |
Environmental factors | Wearables, sensors | Contextual health analysis |
Leveraging Artificial Intelligence in Digital Twin Applications
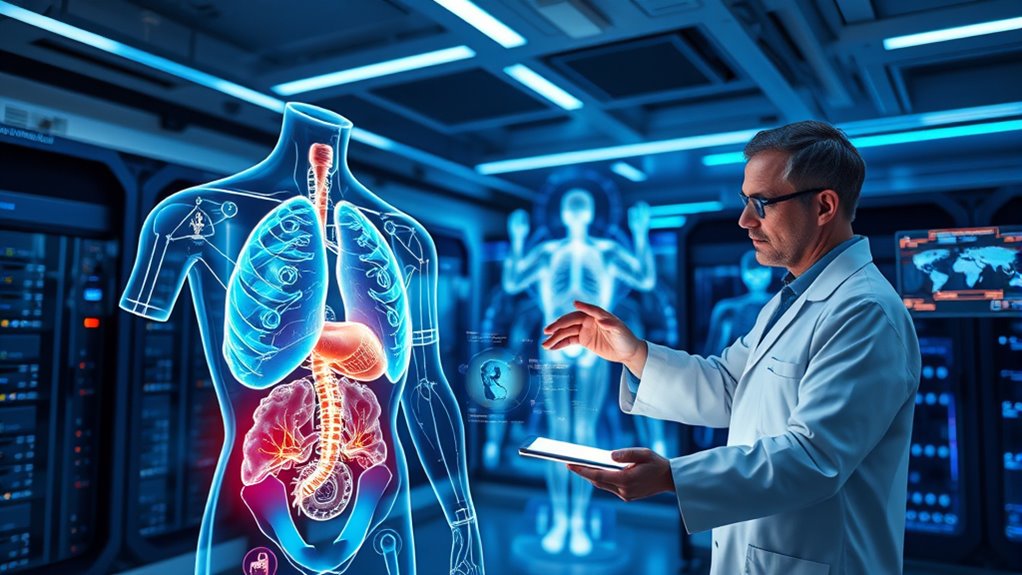
Artificial Intelligence transforms digital twin applications by enabling real-time data analysis, predictive modeling, and autonomous decision-making in personalized healthcare. With AI, you can imagine:
- Virtual models that adapt instantly as new data from genomics, imaging, and wearables flows in.
- Disease simulations that predict health trajectories, allowing early intervention.
- Continuous updates that refine patient-specific models for tailored treatments.
AI algorithms like deep learning and Bayesian networks improve the accuracy of these models, supporting precision medicine.
Overcoming Challenges in Clinical Implementation
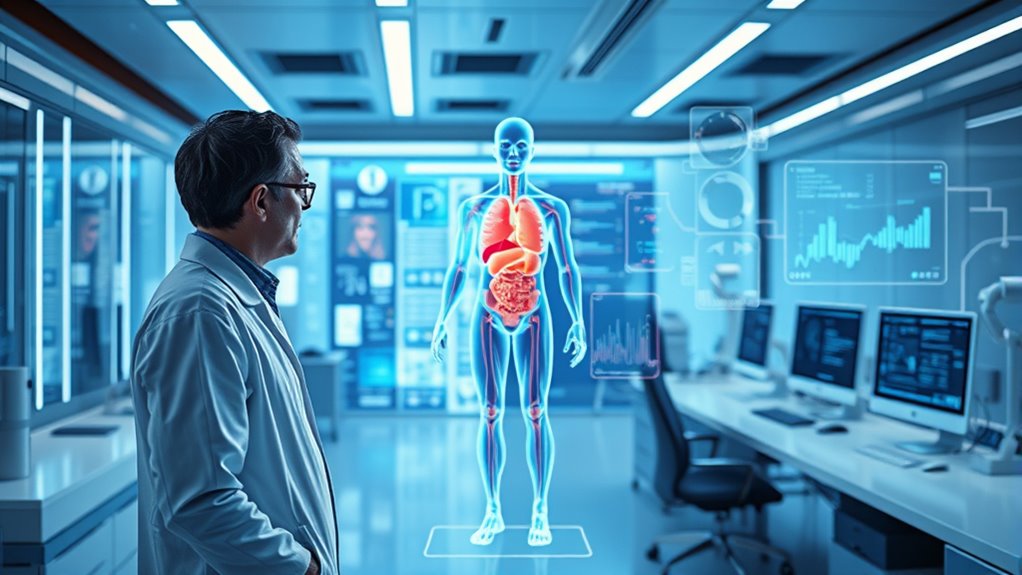
Implementing digital twins in clinical settings presents several significant hurdles that must be addressed to realize their full potential. Achieving interoperability across diverse healthcare systems and data formats is essential for seamless data integration and effective use of multi-omics information.
Developing accurate, real-time models requires overcoming technical challenges related to validation and data quality.
Privacy and regulatory concerns around data ownership and security are critical for building patient trust and ensuring compliance.
User-friendly interfaces are crucial for clinicians and patients to effectively interpret and act on digital twin insights.
Additionally, high costs and resource demands hinder large-scale clinical deployment.
Addressing these challenges involves collaborative efforts to refine technology, establish standards, and prioritize ethical considerations, paving the way for successful integration into routine healthcare.
Future Perspectives and Potential Impact on Medicine
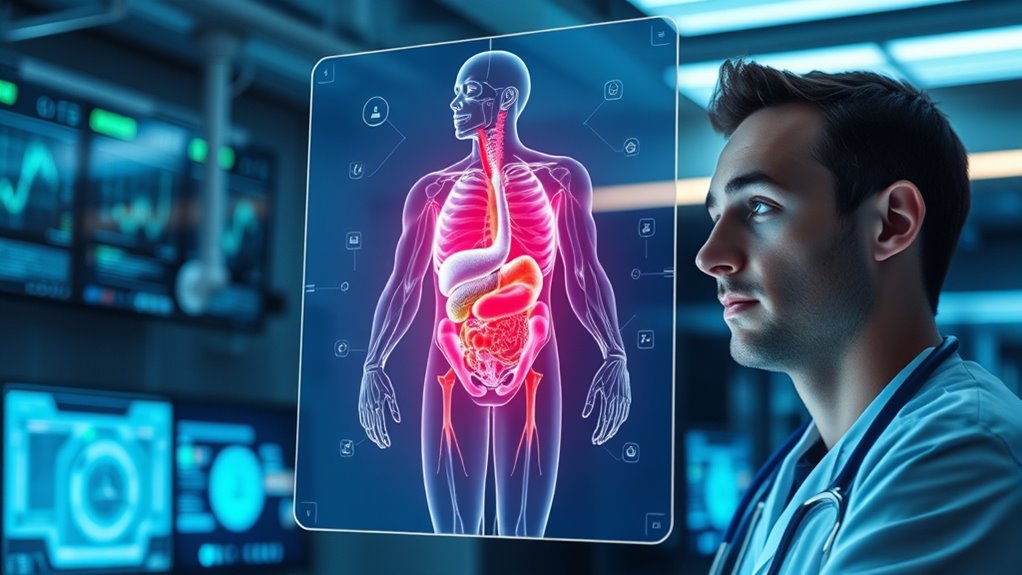
Digital twins are poised to transform medicine by enabling real-time, patient-specific simulations of disease progression and treatment outcomes. This technology paves the way for highly personalized medicine, where predictive modeling guides early interventions and tailored therapies.
Digital twins enable real-time, personalized disease modeling for more precise and effective healthcare interventions.
Imagine:
- Diagnosing diseases with pinpoint accuracy through detailed disease simulation.
- Optimizing treatments by leveraging machine learning and patient-specific data.
- Reducing healthcare costs via improved clinical applications and minimized trial-and-error approaches.
As digital twin technology advances, healthcare innovation accelerates, integrating molecular, cellular, and systemic insights. These models will shape future healthcare, making it predictive, preventative, and personalized.
Overcoming current challenges will open a new era of clinical applications that markedly improve patient outcomes and transform medicine itself.
Collaborative Efforts and Strategies for Advancement
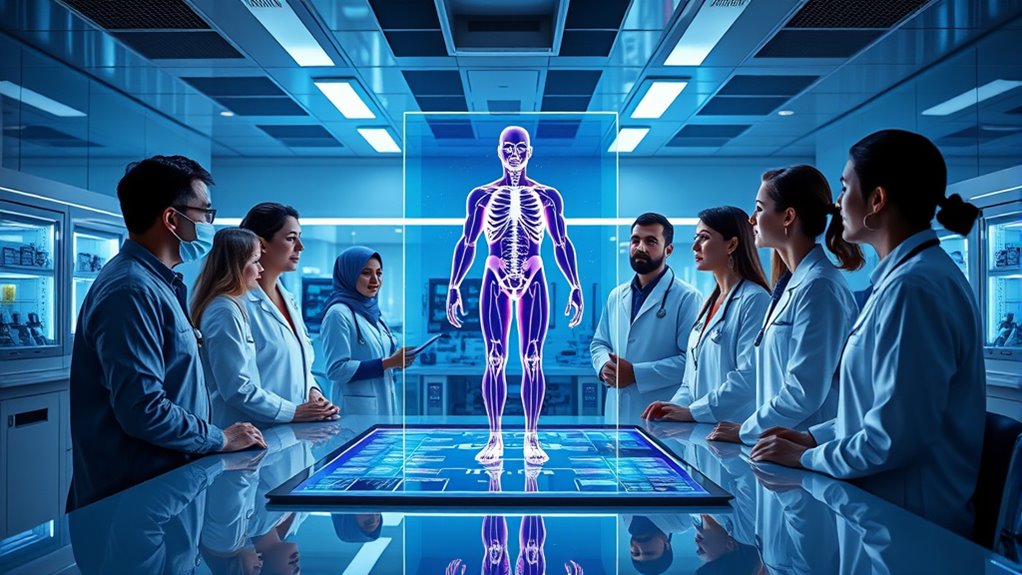
Advancing digital twin technology in healthcare relies heavily on collaborative efforts that bring together diverse expertise and resources. By fostering multidisciplinary partnerships among clinicians, engineers, data scientists, and regulators, you can address complex challenges.
Public-private partnerships, like the European Health Data Space, are crucial for promoting data sharing and resource exchange. Establishing standardization and interoperability protocols ensures seamless integration of varied health data sources, while robust regulatory frameworks safeguard privacy and ethical concerns.
Investing in healthcare infrastructure—such as cloud platforms and IoT sensors—supports real-time data collection and model updates, facilitating clinical adoption. Coordinated strategies that bridge technical, ethical, and regulatory gaps are essential to accelerate progress, enhance interoperability, and maximize the transformative potential of digital twins in personalized medicine.
Frequently Asked Questions
What Are the Digital Twins to Personalized Medicine?
Digital twins for personalized medicine create detailed virtual models of you, combining your genetic, molecular, clinical, and environmental data. They let you simulate disease progression, test treatments, and predict health outcomes in real time.
What Is the Digital Twin for Personal Health?
Think of a digital twin for personal health like a detailed, living map of your body and mind. It’s a virtual model that mirrors your unique physiological, genetic, environmental, and behavioral data.
You can update it in real-time, making it a dynamic tool for understanding your health. This helps you get personalized diagnoses, treatments, and early interventions, ultimately guiding you toward better health outcomes tailored just for you.
What Are the 4 Types of Digital Twins?
You’re asking about the four types of digital twins. Static twins hold only unchanging data, offering a fixed model.
Mirror twins replicate the current state of an object or system but don’t learn or adapt.
Shadow twins gather real-time data to update themselves continuously, enabling self-adaptation.
Intelligent twins go further by using AI and machine learning to analyze, reason, and adapt their behavior autonomously, making them the most advanced.
What Is the Digital Twin Revolution in Healthcare?
Imagine a virtual mirror reflecting your health in real-time, constantly updating with your genetic, molecular, and environmental data. That’s the digital twin revolution in healthcare—you create a detailed, living model of yourself that predicts disease progression and personalizes treatments.
This shift transforms medicine from reactive to proactive, enabling early detection, optimized therapies, and cost savings. Ultimately, it makes healthcare more precise, predictive, and tailored to your unique needs.
Conclusion
As you explore digital twins in healthcare, remember they’re transforming medicine much like the invention of the stethoscope revolutionized diagnosis. By integrating cutting-edge AI and multimodal data, you can personalize care and improve outcomes. While challenges remain, ongoing collaborations and technological leaps promise a future where healthcare is as precise and dynamic as a Swiss watch. Embrace this innovative era, and you’ll be at the forefront of a medical renaissance—no flux capacitor needed.